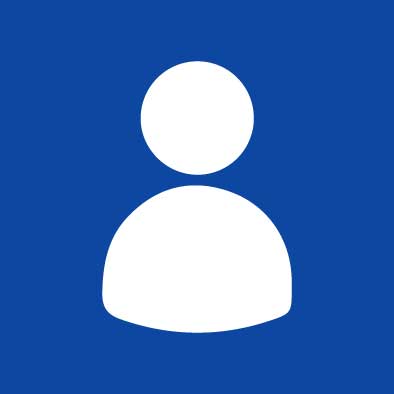
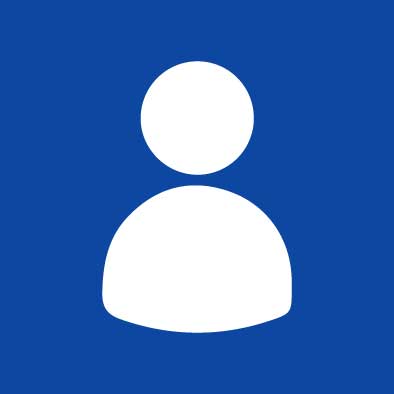
Research area
Abstract
The present interferometric gravitational wave (GW) detectors in the USA (LIGO) and Europe (Virgo) are undergoing a major upgrade, which is targeted to boost the detector sensitivity particularly in the low frequency range. Seismic isolation systems, such as Virgo’s superattenuators, have been developed to suppress the mechanical coupling of seismic motion to unwanted vibrations of the interferometer components. However, fluctuations of the local gravitational field as a result of seismic and atmospheric displacements result in transients which produce Newtonian forces on the interferometer test masses and subsequently inject noise at the interferometer output. These fluctuations are known as Newtonian Noise (NN). Current estimates show that NN will limit the sensitivity of ground based gravitational wave detectors at frequencies around 10 Hz.
Mitigation strategies are under investigation. One possibility is the implementation of Wiener filtering using arrays of seismometers deployed in the vicinity of all test masses. While this approach has been theoretically proven on a semi-infinite terrain slab, its performance is however impaired by the seismic waves interaction with the infrastructure and terrain non-homogeneities. The result is that the seismometers number and placement is rather critical.
Another possibility is to use advanced data analysis techniques (like deep learning) to infer the Newtonian force on the test masses by exploiting a redundant array of non-optimized and mixed sensors (seismometers / tiltometers). The main drawback of this approach is the definition of a sufficiently complex network structure which can incorporate the multiple (unknown) waves interactions.
aThe thesis work is embedded in this effort. It shall start with a series of increasingly complex 3D finite element analysis of the seismic waves / infrastructure interactions to provide the initial dataset used to evolve and train a neural network. The candidate will then use these data into an evolutionary algorithm that looks for the best network matrix. Finally, we shall test the best configuration on real data, as the first series of seismometers are expected to be installed at the beginning of 2020.